The LTV Secrets of Subscription Top Performers
LTV is often misunderstood – learn what it is and how it has a direct impact on your business
Subscription Companies that measure LTV are 8X more likely to be top performers, yet less than 10% of subscription companies measure and manage by LTV.
The relationship between LTV management and company performance is proven. Businesses not currently measuring LTV can quickly improve their business performance by starting to use just one equation and a playbook.
Steve Arentzoff, SVP of Marketing (FlexPay), and Jeffrey Pauletto, Director of Innovation (FlexPay), provide the case for measuring LTV and unlocking the value this equation can provide for your business. They also cover the best practices in operations, KPI management, and organizational structure used by top performing subscription businesses. Even advanced LTV users will learn new insights on how applying LTV to initiatives can extend customer retention and improve growth.
During the 25-minute discussion, our speakers discussed:
- Why LTV Is such a powerful tool to manage subscription businesses
- The barriers to widespread adoption of LTV measurement
- The vision into operational efficiency that LTV provides
- Our recommended equation to measure LTV
- Focused efforts to improve customer retention, and increase LTV
- And more!
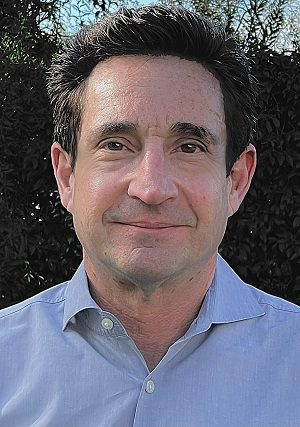
Speaker Bio
Steve Arentzoff
SVP of Marketing, FlexPay
Steve is a modern, results-oriented marketing executive with over 20 years of senior technology marketing leadership, building high-performance marketing organizations, category leadership, marketing and sales alignment, and accelerated company growth rates. Before joining FlexPay, Steve was VP of Demand Generation at Medallia, and Senior Vice President of Global Digital Marketing at Cision.
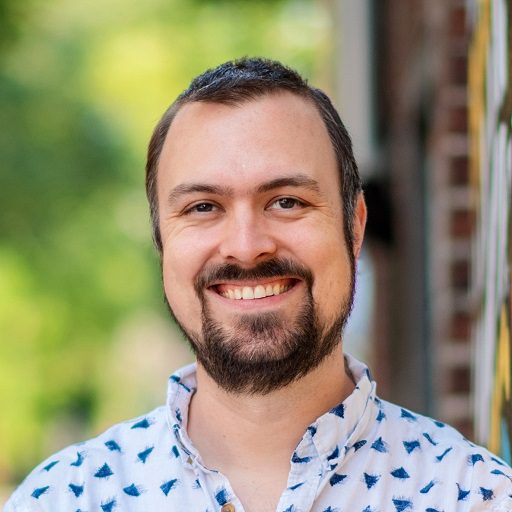
Speaker Bio
Jeffrey Pauletto
Director of Innovation and Data Sciences FlexPay
Jeffrey works with our team of Data Scientists and Data Analysts to produce machine learning models that enhance our understanding of failed payments and maximize recovered revenue.